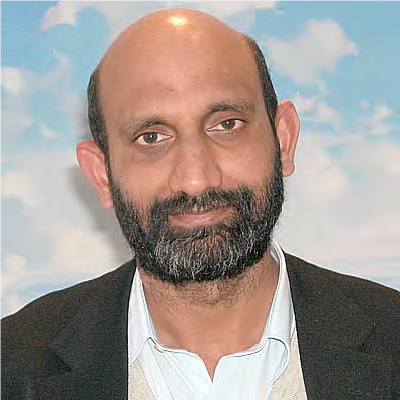
Chennupati Jagadish
- President, IEEE Photonics Society
- President, Australian Materials Research Society
- Distinguished Professor, Australian National University (ANU)
Fields of Specialization: Compound Semiconductor Optoelectronics / Nanotechnology / Photovoltaics / Materials Science
Title: Semiconductor Nanowires for Optoelectronics Applications
Professor Jagadish is a Distinguished Professor and Head of Semiconductor Optoelectronics and Nanotechnology Group in the Research School of Physics and Engineering, Australian National University. He has served as Vice-President and Secretary Physical Sciences of the Australian Academy of Science during 2012-2016. He is currently serving as President of IEEE Photonics Society and President of Australian Materials Research Society. Prof. Jagadish is an Editor/Associate editor of 6 Journals (EIC-Progress in Quantum Electronics), 3 book series and serves on editorial boards of 20 other journals. He has published more than 880 research papers (600 journal papers), holds 5 US patents, co-authored a book, co-edited 11 books and edited 12 conference proceedings and 15 special issues of Journals. He won the 2000 IEEE Millennium Medal and received Distinguished Lecturer awards from IEEE NTC, IEEE LEOS and IEEE EDS. He is a Fellow of the Australian Academy of Science, Australian Academy of Technological Sciences and Engineering, The World Academy of Sciences, US National Academy of Inventors, Indian National Science Academy, Indian Academy of Sciences, Andhra Pradesh Akademi of Science, IEEE, APS, MRS, OSA, AVS, ECS, SPIE, AAAS, FEMA, APAM, IoP (UK), IET (UK), IoN (UK) and the AIP. He received Peter Baume Award from the ANU in 2006, the Quantum Device Award from ISCS in 2010, IEEE Photonics Society Distinguished Service Award in 2010, IEEE Nanotechnology Council Distinguished Service Award in 2011 and Electronics and Photonics Division Award of the Electrochemical Society in 2012, 2013 Walter Boas Medal, 2015 IEEE Pioneer Award in Nanotechnology, 2015 IEEE Photonics Society Engineering Achievement Award, 2016 MRSI Silver Jubilee Anniversary Medal, 2016 Distinguished Fellow of Chinese Academy of Sciences, 2016 OSA Nick Holonyak Jr Award, 2017 Welker Award, 2017 IUMRS Somiya Award and 2017 Nayudamma Award. He has received Australia’s highest civilian honor, AC, Companion of the Order of Australia, as part of 2016 Australia day honors from the Governor General of Australia for his contributions to physics and engineering, in particular nanotechnology. He holds honorary appointments in US, Japan, China and India.
Abstract :
Semiconductor Nanowires are considered as building blocks for next generation electronics and photonics. In this talk, I will discuss about growth of Semiconductor Nanowires using Vapor-Lqiuid-Solid (VLS) and Selective Area Epitaxy (SAE) methods and discuss about materials issues related to nanowires. I will present results on GaAs nanowire lasers, multi-quantum well nanowire lasers, zinc doped GaAs and InP nanowire lasers and nanopositioning of these lasers for flexible electronics applications. I will discuss about use of these nanowires for THz detectors and neuroscience Applications.
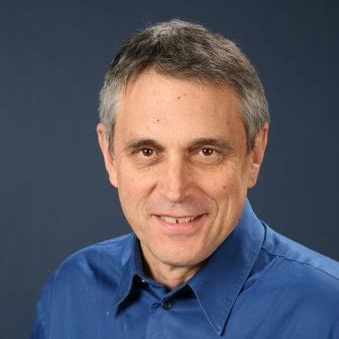
Demetri Psaltis
- Professor and Director, Optics Laboratory, EPFL, Switzerland
Fields of Specialization: Optics / Holography / Imaging / Optofluidics
Title: Learning, neural networks and optics
Demetri Psaltis is Professor of Optics and the Director of the Optics Laboratory at the Ecole Polytechnique Federale de Lausanne (EPFL). He was educated at Carnegie-Mellon University where he received the Bachelor of Science in Electrical Engineering and Economics in 1974, the Master's in 1975, and the PhD in Electrical Engineering in 1977. In 1980, he joined the faculty at the California Institute of Technology, in Pasadena, California where he held the Thomas G. Myers Chair in Electrical Engineering. He served as Executive Officer for the Computation and Neural Systems department from 1992-1996. From 1996 until 1999 he was the Director of the National Science Foundation research center on Neuromorphic Systems Engineering at Caltech. In 2004 he established at Caltech the Center for Optofluidic Integration and he served as the director until he moved to EPFL in 2006 where he established his research lab and served as dean of the engineering school for 10 years. His research interests are imaging, holography, biophotonics, nonlinear optics, and optofluidics. He has over 400 publications in these areas. Dr. Psaltis is a fellow of the IEEE, the Optical Society of America, the European Optical Society and the Society for Photo-optical Systems Engineering (SPIE). He received the International Commission of Optics Prize, the Humboldt Award, the Leith Medal, the Gabor Prize and the Joseph Fraunhofer Award/Robert M. Burley Prize.
Abstract :
Learning to perform various tasks by training neural networks has been linked to optics for a long time [1]. The remarkable progress that has been achieved in recent years with “deep learning” networks, has led to new many ideas for how to use learning techniques in the design and operation of optical systems [2,3,4,5] and vice-versa [6]. We will present results from this recent activity with particular emphasis of how deep neural networks can enhance the capabilities of optical imaging systems.
[1] Abu-Mostafa, Yaser S., and Demetri Psaltis. “Optical Neural Computers.” Scientific American, vol. 256, no. 3, 1987, pp. 88–95., www.jstor.org/stable/24979343.
[2] U. Kamilov, I. Papadopoulos, M. Shoreh, A. Goy, C. Vonesch, M. Unser, and D. Psaltis, "Learning approach to optical tomography," Optica 2, 517-522 (2015).
[3] Ayan Sinha, Justin Lee, Shuai Li, and George Barbastathis, "Lensless computational imaging through deep learning," Optica 4, 1117-1125 (2017)
[4] Rivenson, et. al. , “Phase recovery and holographic image reconstruction using deep learning in neural networks”, Light Science and Applications 7,17141, Feb 2018
[5] N. Borhani, E. Kakkava, C. Moser, and D. Psaltis, "Learning to see through multimode fibers," Optica 5, 960-966 (2018).
[6] Shen, et. al, “Deep learning with coherent nanophotonic circuits”, Nature Photonics, 11 (2017).
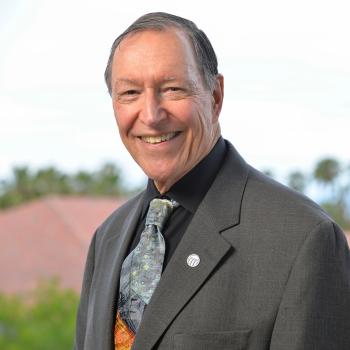
James S. Harris
Fields of Specialization: Molecular Beam Epitaxy / Nanofabrication / Optoelectronics / Non-linear Optics / Single Electron Devices / Spintronics
Title: The Acceleration of Electrons with Light in Semiconductor Nanostructures
Harris utilizes molecular beam epitaxy (MBE) of III-V compound semiconductor materials to investigate new materials for electronic and optoelectronic devices. He utilizes heterojunctions, superlattices, quantum wells, and three-dimensional self-assembled quantum dots to create metastable engineered materials with novel or improved properties for electronic and optoelectronic devices. He has recently focused on integration of photonic devices and micro optics for creation of new minimally invasive bio and medical systems for micro-array and neural imaging, a retinal prosthesis, high efficiency solar cells and solar to hydrogen generation and laser arrays and single photon detectors for LIDAR systems.
Abstract :
High energy particle beams and their generation of X-rays have been foundational elements in physics research the past 7 decades and medical therapy. The acceleration is achieved by high power radio frequency (RF) fields. Unfortunately, their widespread availability is seriously limited by their enormous size and huge cost, creating a strong need for alternatives. Over the past 7 years, a new breed of accelerator nanotechnologists using technologies driven by advances in semiconductor fabrication and “Moore’s Law” have now demonstrated several KeV acceleration on micron scale dimensions using high peak power lasers in dielectric nano-structures—a Dielectric Laser Accelerator (DLA)–whose near fields synchronously accelerate charged particles. To realize a practical “accelerator on a chip”, we are developing an integrated system with several crucial elements: dielectric nano-structures serve as phase masks for sustained energy gain, self-focusing structures to keep the beam well collimated, waveguides for efficient energy coupling and dielectric “undulators” for the generation of X-rays.
We have demonstrated these essential elements with sub-relativistic electron beams and describe progress in laser electron acceleration with silicon dual-pillar grating structures with record high acceleration gradients [1] and transverse self-focusing of the electron beam [2].
[1] Leedle, K., et. al., Optica 2 158-161 (2015).
[2] Leedle, K. J. et al. Opt. Lett. 40, 4344 (2015).
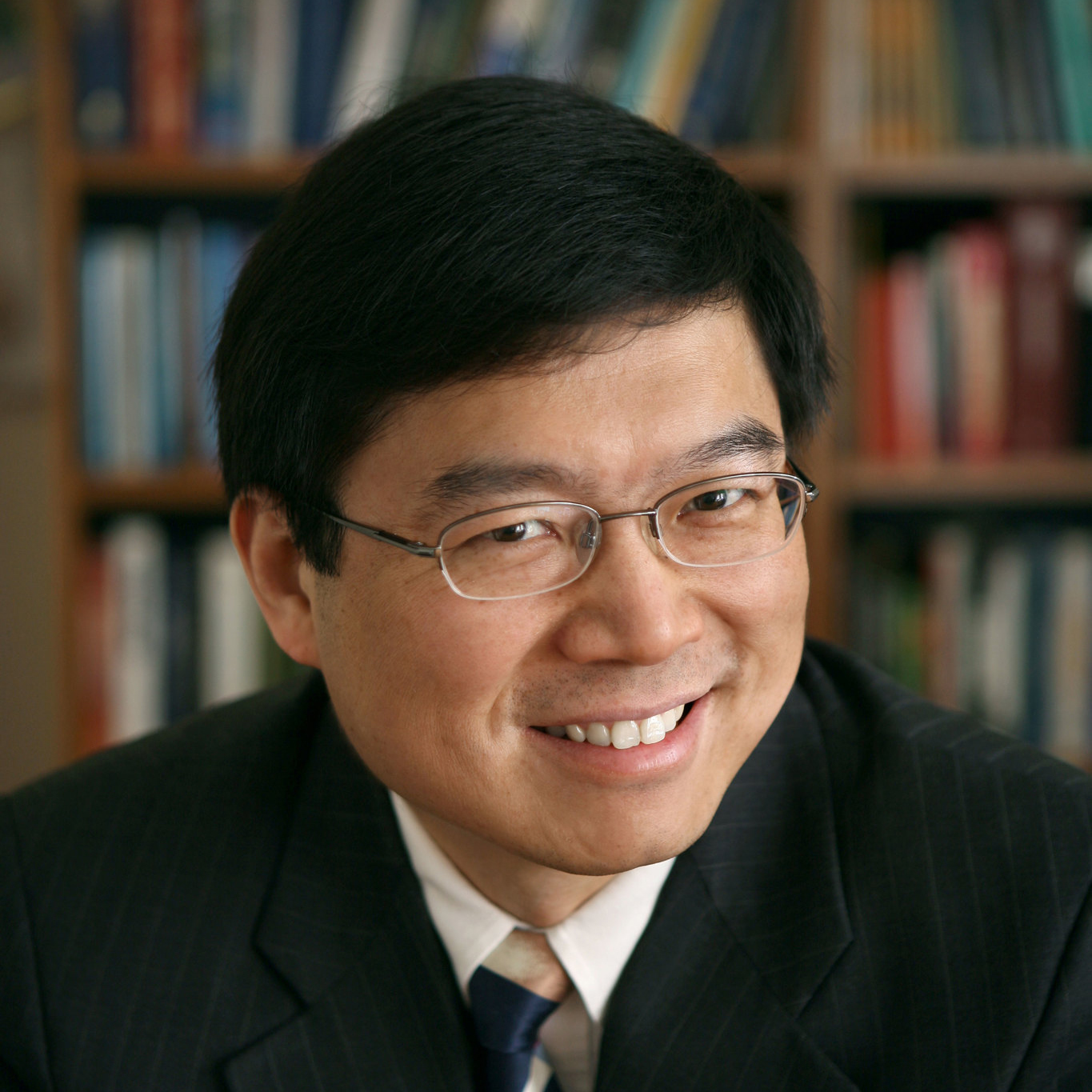
Lihong Wang
- Professor, Medical Engineering and Electrical Engineering, CalTech
Fields of Specialization: Biomedical Imaging / Photoacoustic Imaging
Title: World’s Deepest-Penetration and Fastest Optical Cameras: Photoacoustic Tomography and Compressed Ultrafast Photography
Lihong Wang earned his Ph.D. degree at Rice University, Houston, Texas under the tutelage of Robert Curl, Richard Smalley, and Frank Tittel. He is Bren Professor of Medical Engineering and Electrical Engineering at California Institute of Technology. His book entitled “Biomedical Optics: Principles and Imaging,” one of the first textbooks in the field, won the 2010 Joseph W. Goodman Book Writing Award. He also edited the first book on photoacoustic tomography and coauthored a book on polarization. He has published 470 peer-reviewed articles in journals, including Nature (Cover story), Science, PNAS, and PRL, and has delivered 460 keynote, plenary, or invited talks. His Google Scholar h-index and citations have reached 117 and 57,000, respectively. His laboratory was the first to report functional photoacoustic tomography, 3D photoacoustic microscopy, photoacoustic endoscopy, photoacoustic reporter gene imaging, the photoacoustic Doppler effect, the universal photoacoustic reconstruction algorithm, microwave-induced thermoacoustic tomography, ultrasound-modulated optical tomography, time-reversed ultrasonically encoded optical focusing, nonlinear photoacoustic wavefront shaping, compressed ultrafast photography (10 trillion frames/s, world’s fastest camera), Mueller-matrix optical coherence tomography, and optical coherence computed tomography. In particular, photoacoustic imaging broke through the long-standing diffusion limit on the penetration of optical microscopy and reached new depths for noninvasive biochemical, functional, and molecular imaging in living tissue at high resolution. He chairs the annual conference on Photons plus Ultrasound, the largest conference at Photonics West. He was the Editor-in-Chief of the Journal of Biomedical Optics. He received the NIH’s FIRST, NSF’s CAREER, NIH Director’s Pioneer, and NIH Director’s Transformative Research awards. He also received the OSA C.E.K. Mees Medal, IEEE Technical Achievement Award, IEEE Biomedical Engineering Award, SPIE Britton Chance Biomedical Optics Award, Senior Prize of the International Photoacoustic and Photothermal Association, and OSA Michael S. Feld Biophotonics Award. He is a Fellow of the AIMBE, Electromagnetics Academy, IEEE, OSA, and SPIE. He was inducted into the National Academy of Engineering. An honorary doctorate was conferred on him by Lund University, Sweden.
Abstract :
We developed photoacoustic tomography to peer deep into biological tissue. Photoacoustic tomography (PAT) provides in vivo omniscale functional, metabolic, molecular, and histologic imaging across the scales of organelles through organisms. We also developed compressed ultrafast photography (CUP) to record 10 trillion frames per second, 10 orders of magnitude faster than commercially available camera technologies. CUP can tape the fastest phenomenon in the universe, namely, light propagation, and can be slowed down for slower phenomena such as combustion.
PAT physically combines optical and ultrasonic waves. Conventional high-resolution optical imaging of scattering tissue is restricted to depths within the optical diffusion limit (~1 mm in the skin). Taking advantage of the fact that ultrasonic scattering is orders of magnitude weaker than optical scattering per unit path length, PAT beats this limit and provides deep penetration at high ultrasonic resolution and high optical contrast by sensing molecules. Broad applications include early-cancer detection and brain imaging. The annual conference on PAT has become the largest in SPIE’s 20,000-attendee Photonics West since 2010.
CUP can image in 2D non-repeatable time-evolving events. CUP has a prominent advantage of measuring an x, y, t (x, y, spatial coordinates; t, time) scene with a single exposure, thereby allowing observation of transient events occurring on a time scale down to 100 femtoseconds, such as propagation of a light pulse. Further, akin to traditional photography, CUP is receive-only—avoiding specialized active illumination required by other single-shot ultrafast imagers. CUP can be coupled with front optics ranging from microscopes to telescopes for widespread applications in both fundamental and applied sciences.
Selected publications
- Nature Biotechnology 21, 803 (2003).
- PRL 92, 033902 (2004).
- Nature Biotechnology 24, 848 (2006).
- Nature Protocols 2, 797 (2007).
- Nature Photonics 3, 503 (2009).
- Nature Materials 8, 935 (2009).
- Nature Photonics 5, 154 (2011).
- Nature Materials 10, 324 (2011).
- Science 335, 1458 (2012).
- Nature Medicine 18, 1297 (2012).
- PNAS 110, 5759 (2013).
- PNAS 111, 21 (2014).
- Nature 516, 74 (2014).
- Nature Photonics 8, 931 (2014).
- Nature Photonics 9, 126 (2015).
- Nature Communications 6, 5904 (2015).
- Nature Methods 12, 407 (2015).
- Nature Methods 13, 67 (2016).
- Nature Methods 13, 627 (2016)
- Science Advances 3, e1601814 (2017)
- Nature Biomedical Engineering 1, 0071 (2017)
- Science Advances 3, e1602168 (2017)
- Nature Communications 8, 780 (2017)
- Nature Communications 8, 1386 (2017)
- Nature Communications 9, 2352 (2018)
- Nature Communications 9, 2734 (2018)
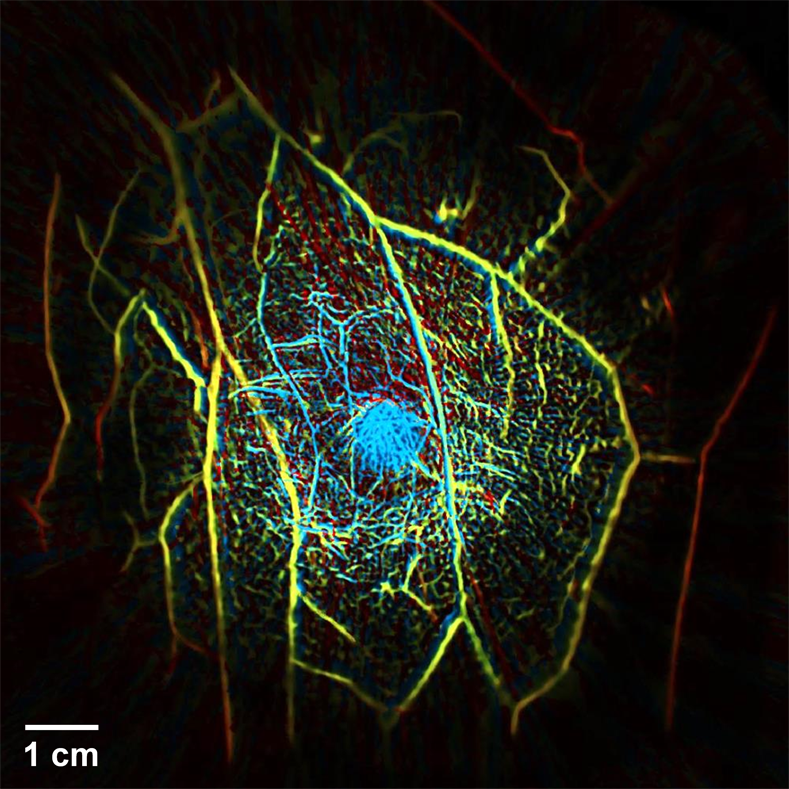
In vivo 3D photoacoustic tomography of a human breast
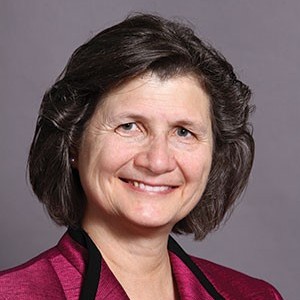
Maryellen L. Giger
- President, SPIE
- A. N. Pritzker Professor of Radiology / Medical Physics, The Univ. of Chicago
Fields of Specialization: Computer-aided Diagnosis / Quantitative Image Analysis (Radiomics) / Machine Learning (Deep Learning)
Title: Machine Learning in Breast Cancer Diagnosis and Management
Maryellen L. Giger, Ph.D. is the A.N. Pritzker Professor of Radiology, Committee on Medical Physics, and the College at the University of Chicago. She is also the Vice-Chair of Radiology (Basic Science Research) and the immediate past Director of the CAMPEP-accredited Graduate Programs in Medical Physics/ Chair of the Committee on Medical Physics at the University. For 30 years, she has conducted research on computer-aided diagnosis (radiomics) and machine learning in the areas of breast cancer, lung cancer, prostate cancer, and bone diseases. She has also served on various NIH study sections, is a former president of the American Association of Physicists in Medicine, was the inaugural Editor-in-Chief of the SPIE Journal of Medical Imaging, and is the current President of SPIE. She is a member of the National Academy of Engineering, a Fellow of AAPM, AIMBE, SPIE, and IEEE, a recipient of the AAPM William D. Coolidge Gold Medal and the EMBS Academic Career Achievement Award, and is a current Hagler Institute Fellow at Texas A&M University. She has more than 200 peer-reviewed publications (over 300 publications), has more than 30 patents and has mentored over 100 graduate students, residents, medical students, and undergraduate students. Her research in computational image-based analyses of breast cancer for risk assessment, diagnosis, prognosis, response to therapy, and biological discovery has yielded various translated components, and she is now using these image-based phenotypes in imaging genomics association studies.